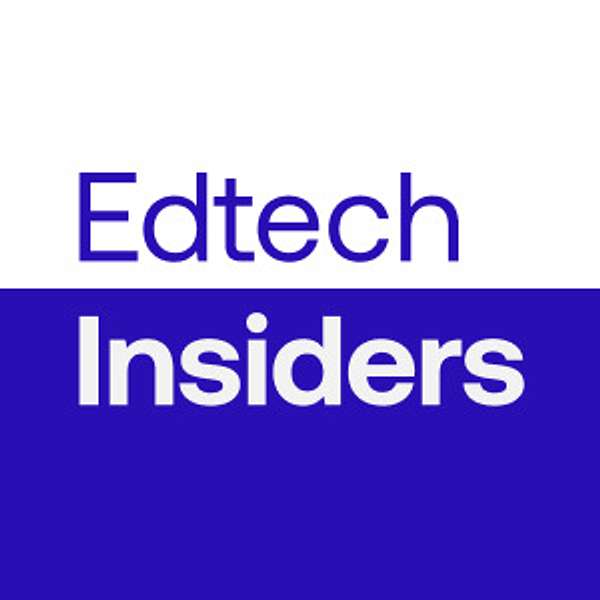
Edtech Insiders
Edtech Insiders
Week in Edtech 8/22/2024: OpenAI's Project Strawberry, Google’s Gemini Live, Sal Khan at EduTECH 2024, California Partners with NVIDIA, MIT's AI Risk Database and More! Feat. Dr. Steve Ritter of Carnegie Learning and Christopher Kahler of Kinnu
Join Alex Sarlin and Ben Kornell, as they explore the most critical developments in the world of education technology this week:
🎯 Rumors about OpenAI's "Project Strawberry"
📰 ChatGPT now includes Wired, Vogue, and The New Yorker
🤝 New AI fund by GitLab Foundation, Ballmer Group, and OpenAI
🔊 Google launches Gemini Live to rival ChatGPT
👨🏫 Sal Khan says teachers matter more than tech at EduTECH 2024
🤖 California partners with NVIDIA to enhance AI education
⚠️ MIT releases a comprehensive AI risk database
📉 Softbank-backed Paper cuts 45% of head office jobs
🚀 Go1 CEO change and IPO plans ahead
Plus special guests:
- Dr. Steve Ritter, Founder and Chief Scientist at Carnegie Learning
- Christopher Kahler, Co-founder and CEO at Kinnu
Stay updated with the latest Edtech news and innovations. Subscribe to Edtech Insiders podcast, newsletter and follow us on LinkedIn!
This season of Edtech Insiders is once again brought to you by Tuck Advisors, the M&A firm for EdTech companies. Run by serial entrepreneurs with over 25 years of experience founding, investing in, and selling companies, Tuck believes you deserve M&A advisors who work as hard as you do.
Welcome to EdTech insiders, the top podcast covering the education technology industry from funding rounds to impact AI development across early childhood, K 12, higher ed and work, you'll find it all here at edtech Insider.
Ben Kornell:Remember to subscribe to the pod. Check out our newsletter and also our event calendar. And to go deeper, check out edtech insiders, plus where you can get premium content. Access to our WhatsApp channel, early access to events and back channel insights from Alex and Ben. Hope you enjoyed today's pod. Hello, edtech insiders, it is back to school time. I just dropped off my kids at school this morning, Alex, your young one and soon to be are still not school age yet, but they're going to preschool. Everybody's back into the fall rat race. Sometimes it just actually feels good to get back into the routine. So welcome back, edtech insiders. For those of you who are back into your week in edtech routine, I'm Ben Kornell, and this is Alex sarlin, your host for edtech insiders, welcome.
Alexander Sarlin:Hey, great to see you. Ben, I know we've had an exciting summer trying to keep everything moving in this sort of quiet period where people are in and out of the office and everybody's prepping for September. Here we are, end of August, things are really getting started. Yes, my son is on his second week of preschool, and he is still very sad each day when we leave, so we're hoping that we can sort of quell that separation anxiety. He's such a sweetheart, and it's, you know, Paul's the heartstrings. I know a lot of our listeners have been through this, but it's my first time, so it's tricky. What is going on on the events front well?
Ben Kornell:So speaking of heartstrings, we are so excited to see all of you. Our first event of the season is September 18 in San Francisco. We hope you can make it four to 6:30pm at the EdTech insiders happy hour. And then we'll also be at New York edtech week on Tuesday the eighth, with the magic edtech rooftop party. So grateful to have such great sponsors, reach capital and match, get tech as well as our presenting sponsors, Cooley and tuck advisors. So we hope that you can make it out to either of those events, and of course, check out the newsletter and pod. So who do we have coming up on the pod? Yeah. So
Alexander Sarlin:week, we are getting our interview with Paul this LeBlanc from snoo out. I've been promising this one for the last couple of week at edtechs, but it is truly here. So check that one out. Paul LeBlanc, obviously a legend, sort of, maybe as close as we get to a, almost like a, I know, dare I say, like a Charles Barkley. Is that a weird metaphor of edtech, somebody who's just a universally acclaimed I don't want to say to Michael Jordan, I don't like Michael Jordan, but I'm gonna say Charles Barkley. I had a really interesting interview with Oliver page. He's the CEO of a company called cybernet, and he actually comes to the EdTech space from hardware and from cybersecurity. It's two areas we almost never cover on the podcast, and it was a really interesting conversation. Keep your eye out for all sorts of things coming this month. We have two amazing guests this week. We're talking at the end of this episode to Steve Ritter, the CEO and founder of Carnegie, learning an absolutely epic, legendary ed tech company, very much based in evidence and efficacy. And we're talking to Chris collar, the CEO and co founder of Kinnu, which is one of my personal favorite apps. It's all about learning and memorizing, but it really, really understands how people learn, and they're using a very interesting method for product development through learning efficacy. All right, let's get into some of the news. What jumped out to you this week?
Ben Kornell:Well, let's kick off with AI. The AI beat has been full. There's a ton of new news, but in terms of what's most important for edtech, what we saw is a bunch of rivaling dueling announcements between open AI and Google. I'll go with the Google ones. Maybe you can cover some of the open AI ones on the Google front, I got to spend a whole day with the learnx team at the Googleplex. There's an entire building, which, you know, started with the Apps for Education, and now it has grown to an entire cross functional team, learnx. Led by Ben Gomes, who used to lead search, really thinking about learning, not just education, across all of their surfaces. And so there's just been an incredible drum beat around Gemini. And I think the compelling through line here is, you know, they're bringing AI embedded to wherever you are, whatever apps you're using. This week, some of the big announcements have been around Android and the mobile phone and AI enablement and AI assistance. There, what we're seeing is basically a conversational AI assistant in your hands, a la chatgpt app, but embedded into all the functions that you need, like opening an email or creating a calendar appointment. We also are seeing a bunch of these features that are migrating to Google Classroom as part of the back to school push. So they just celebrated their 10 year anniversary, and it felt like a rejuvenated group of like we are actually building new products, new features, new capabilities. The big one, I think, is the quizzes. So assessment has been a largely missing element of Google Classroom, but now the ability for teachers to create quick quizzes and quick assessments is all in there, and I think it does speak to the strategy of Google, which is teachers are still the primary user here, and really enabling the teacher to use the AI for their purposes, seems to be the kind of key education strategy, whereas, you know on the student side, that's going to be much more on consumer facing tools like YouTube and Google Docs and G Suite, et cetera. How about on OpenAI front? Yeah, so
Alexander Sarlin:OpenAI had a number of different sort of announcements or even rumors this week. One announcement that I found very interesting is OpenAI is working with the GitLab Foundation and the Balmer group. We recognize Steve Ballmer as you know, ex Microsoft GitLab Foundation. We talked to Ellie bertani, who's the head of the GitLab Foundation, on the podcast a while back, and they are doing really interesting things for tech and equity, and basically the three are all working together to launch a new fund to foster AI innovation. It's very interesting, and they're offering grants for high potential projects. So definitely worth keeping an eye on. We also saw OpenAI continue to expand its partnerships. So they just signed a multi year content partnership with Conde Nast that gives them access to content from Wired Magazine, Vogue, The New Yorker and many other magazines. OpenAI has been continuing to do this sort of crawling through the web of human knowledge after they've already scraped as much as they can off the internet and trying to make all of these content deals. What that's going to mean for open AI's tools or their strategy is yet to be seen. But it is definitely interesting to have access to the archives of everything ever written in The New Yorker or wired in terms of tech and literature and culture. It's just interesting. We'll see where it all goes. And then the third is, there's a rumor right now about, you know, we talked a little bit about this in the last couple weeks, but this concept of project strawberry, they're calling it basically, you know, everybody's always curious about, is anybody taking a leap forward in this space? Is anthropic doing it? Is OpenAI doing it? Is Google doing it, and it feels like there's some really interesting rumors about a sort of reasoning focused AI, something that goes deeper into actual sort of mental, critical reasoning beyond what we've seen so far. We're also continuing to see arms races around video. So we saw Nvidia. It has a video foundational model that they've trained on, you know, years and years and years and years of video and open AI's Sora, which they announced a while back, is still sort of inching its way towards fruition and release and runway, which is a sort of an earlier player in the real time AI video space. They just have a new model called Gen three turbo that can basically make AI videos in seconds. And you know, you've heard me say this 100 times on the podcast, Ben, but the age of AI video is so near, I just don't think we're appreciating it enough. We're still talking about text, we're still talking about voice. And yes, these things are huge. They're going to be important. But AI video, I mean, when the Internet became video capable, it was a sea change. And I think we're really close. Yeah,
Ben Kornell:I think that the narrative in education, which has been all about the excitement of what AI can do, we've definitely we're going through the kind of hype cycle curve, and now we're starting to climb the ladder of functionality. But these breakthroughs, and specifically breakthroughs with reasoning and breakthroughs with modality actually make the practical use cases far more compelling. It's interesting. So kind of bringing this to the education space, one of the big headlines this week was an interview with Sal Khan, who were actually doing the book club about Sal Khan. Brave New World Book, and the messaging is a big contrast to original messaging, which was, AI is going to transform education in five years. We are not going to recognize, you know, schools as they are today. And I think it's, you know, Sal's messaging has shifted to this is going to be about teachers first and educators, and integrating with schools and systems and finding ways to support them, and a much more pragmatic, practical approach around where can AI add value? I actually find this to be like a healthy intersection where we're heading now here this fall, I think there's like a rational optimism that was, you know, before it was this over exuberance and skepticism and swinging back and forth, but I think we've got enough momentum to show that some of the efficiency pieces are playing out and some of the potential around things like assessment, around nudging or prompting or practice and engagement. It's not a full tutor at this point, but this idea of assistive AI is coming to fruition in like, small but meaningful ways. And so you know, for those of you who want to check that out, the Sal Khan interview. You can check it out, and got it from the ASU GSV email letter, but you know, he's been, in some ways, the figurehead of our movement, and it's in the education hq.com website. It's just a fascinating read.
Alexander Sarlin:I think, you know, we're going to go through so many phases of like these sort of mini hype cycles in AI, around specific aspects of it, like tutoring or video or reasoning or AGI or their hype cycles. And there's this, I'm writing a piece for the newsletter right now about moral panics. Right like, you know, the potential for moral panic around AI, around so many different aspects of AI is so huge. And if we look back, you know, to the early days of the internet and all the different moral panics that have come in the, you know, two decades there, I think it's going to be a really interesting roadmap for that. And I think Khan is probably trying to get ahead of the inevitable, I won't say moral panic, but the inevitable backlash and fear that comes with AI tutoring. And one of the messages in this interview is really about teachers coming first, right? Because I think that's it's easy to extrapolate out from the concept of AI tutoring that there's a threat to the role of educators and to tutors. And I think he's trying to make sure that that is not how this lands on the higher ed front. Interesting announcement this week. So you know, this came out August 14, so just a little bit over a week ago, we Gavin Newsom has been really trying to lean into AI. He's the governor of California, a big and a national figure in politics in the US, 35 of the top 50 companies in AI are in California, you know, mostly in Northern California, as we know, Nvidia, arguably the biggest AI company out there, as well as open AI and Google. And all the ones we talking about are almost all in California. And he's actually trying to foster a really nice relationship between AI companies, especially Nvidia, and the community college system, which is the biggest in in the country, and absolutely enormous system. You know, in this they just signed this, and Nvidia is basically going to create AI laboratories, develop curricula, certifications, and try to integrate AI really, into a lot of the Associate Degrees and professional development tools that are happening in the community college level. That's really exciting, and it's really interesting to think about, because, you know, community colleges, because they are serving a different population than, certainly than selective colleges sometimes are really more agile and sort of able to move faster, and because they have a, often a sort of deeper connection with the government in a way like they could be sort of swept up in movements. I'm really excited about this. I can imagine a world. And we talked to Georgia Tech, and Georgia Tech had this amazing Nvidia sponsored, you know, AI Lab. They're doing really cool things there. Georgia Tech is one of the very best tech schools in the country. Very few people get the chance to go to Georgia Tech, if the community college system of California gets to do AI in this big way, you and I both know Claire Fisher, who does AI innovation there. You know, I think we're doing something with AI that maybe allows for a sort of leapfrog moment for tech, where people can access this new technology and the certifications around it in a way that is a that may actually go faster than, say, traditional liberal arts schools. I'm curious, do you think that this is just, you know, is it just hand wavy? Is it just politics? Or do you feel like this kind of deal between Nvidia and California community colleges might be something we look back on and say, Oh, wow. That was one of the, you know, the first moments where we really started to see AI and education getting really close together.
Ben Kornell:I have a couple different takeaways, and it's hard to ever say this moment or that moment is going to be a true inflection point. It feels. Still more like a drum beat of events. What I'm seeing, though, is that AI education, so learning about AI and how to do AI and how to use AI, how to code with AI, is the best space for people to implement AI powered tools, because it's meta, you know, here, working in AI, learning about AI with AI. And so it actually is the most successful, adaptive, welcoming place for an AI enablement. You actually saw Andre kaparthie, the former open AI. He's building a personal AI tutor. But if you read the small print, it's an AI tutor to teach AI. And so I think this is an area where anyone focused on career education is going to be out ahead of traditional schooling. And that's where community colleges are a great, great fit. So I love and you and I, we kind of like the people who are on the edge of what's possible, and so almost always career and professional, you know, programs are just more innovative than you're going to have in your traditional K 12 or university systems. I think that this is an area where there are some leap forward possibilities. Now I will also say we have to remember that many of these systems are unionized. Many of these systems are resistant to corporate interloping in what is viewed as like a, you know, a government public sector delivery mechanism. And so I expect us to also see tensions around capitalism, around AI and its advancement, with like workers rights and people feeling like their roles are being replaced or being threatened, and this will be the tip of the spear if Nvidia and California Community Colleges doesn't raise those concerns, I would be shocked that to me, I think is kind of a leading indicator of how AI is going to work in education is looking at these, you know, learn AI courses.
Alexander Sarlin:That's a really good take. And I love the idea of it being sort of a drumbeat of things that are being tried, some of which will will continue to drum, and some of which we may fade out. And yes, AI education. It feels like a place a lot of people are jumping in. And AI education for workforce development, especially, I think this is one to keep an eye on, because you're right, there could be a major pushback. This could be one of these areas where corporate and public sector actually really clash. Or it could be a great, you know, public private partnership we always want to see. But before we get to our guests, just two little pieces of, you know, I think worthwhile edtech scuttlebutter, not scuttlebutt. Actual things that are happening. We saw two CEO swaps this week from pretty well known edtech companies, paper. We've been talking about paper for a while. Paper has been in a lot of trouble for quite a while. Cut a number of jobs again this week and fully replaced Philip Cutler, their founder. That's sort of been a long time coming. We got to interview him on the show a year ago, but post pandemic, or he's not even post pandemic, post Esser, I think paper has really struggled a lot, and there's going to be some changes there, and then go one, which is the Australian unicorn, that's sort of a workforce development company, also swapped their CEO, but for somewhat different reasons, they are starting to talk about ipoing, which is exciting. We have not heard about ad tech IPOs you know very much recently. So that's a good one to keep in mind. That's out in Australia. Any thoughts on those before we jump to our guests? Ben, well, I
Ben Kornell:think, you know, Oppenheimer released their report. So this, this is actually quite timely on the IPO and the TLDR on it is that we're just seeing a spike in M A and when you look at their deck, the number of transactions are pretty astounding. At the same time, it's all overshadowed by the Power School deal and the Instructure deal. Instructure deal has not closed yet, but those are the ones that have publicly available data, that provide comms that are in the billions of dollars, but there's just a ton of M and A activity on the venture front, the numbers are stark. Number of new investments, incredibly low. Number of double down investments, incredibly high. There's a three to one ratio of doubling down investments, people investing in existing companies that they're already in their portfolio versus new companies. So I think we're seeing a big shift in the venture space, which was chasing new deals now, it's all about, you know, writing your winners and, you know, in a worst case scenario, saving those investments that need to get through a tough time and then on the public markets. Just to all of the listeners, I would say, Please don't read that section. It. Is bloody. It is depressing. It's like a very, very gory war story. And the only like points of light are in some higher ed workforce sector places. But the order of magnitude of those wins versus the order of magnitude, you know, even Duolingo is down. I mean, when Duolingo is down, you're like, Oh, crap. And so I do think that that creates a downward pressure on valuations, which then feeds back into point number one, which is M and A is on fire right now, because valuations are now viewed as reasonable and private equity capital is active.
Alexander Sarlin:It's validating. We've predicted that, you know, a long time ago, and then it didn't feel like it was happening, but it seems like little bit delayed, but it is happening. It's made sense. I mean, it makes sense that in this, you know, wintery, odd environment where people cannot get funding on less like you say they're already, they're already well funded, then you know, there's a lot of room for acquisition. I think there may be another whole wave about some of these AI companies, because, you know, the acquisitions now are sort of big people getting medium sized, but there's a ton of this fast moving, tiny AI companies that are going to have to find a home or or shut down. And I think we may see, you know, a number of consolidations there as well. I have not read the Oppenheimer report yet. I'm really excited to read it and talk to you about it more next week on this show. Let's jump to our guests for the week. We have amazing guests this week.
Ben Kornell:Today we are joined by Steve Ritter of Carnegie learning. Steve is the founder and chief scientist of Carnegie learning, a global leader in artificial intelligence for K 12 education. And Dr Ritter is a pioneer in the education technology industry, developing the first AI powered math curriculum over 25 years ago, Dr Ritter earned a doctorate in cognitive psychology at Carnegie Mellon University, and was instrumental in the development and evaluation of the cognitive tutors for mathematics. He led the transfer of the cognitive tutor technology to Carnegie learning, where it forms the basis of the company's award winning mafia intelligent tutoring systems, the only curriculum to achieve a perfect score by independent nonprofit Ed reports. The author of numerous papers on the design, architecture and evaluation of adaptive instructional systems, Dr Ritter is recognized as an expert on the design and evaluation of education technology and on educational analytics. He is lead author of an evaluation that is one of the few to be judged by the US Department of Education's What Works Clearinghouse as meeting their standards without reservations, the winner of prestigious awards, including the best paper award at the International Conference on educational data mining. He continues to speak across the globe at conferences on the future of AI in education technology at Carnegie learning, Dr Ritter leads a research team devoted to using learning engineering to improve the efficacy of the company's products. Current funding on research projects under his leadership exceeds $90 million from the federal government and major foundations such as gates, Walton Schmidt, futures, etc, in partnership with top universities, his team is focused on such issues as algorithmic bias in educational AI supports for Teaching Math to struggling readers, and The upgrade tool for supporting rigorous field tests of educational software. A little bit about Carnegie learning before we dive in Pittsburgh based Carnegie learning is at the forefront of ed tech companies using data and AI to dramatically improve learning outcomes for students, a leader in K 12 education for 26 plus years, Carnegie's award winning math literacy, world languages, professional learning and high dosage tutoring. Products are used by over 5.5 million students and educators in all 50 states and Canada. And as I said, before Carnegie learning was born out of Carnegie Mellon University, one of the founding universities of many edtech companies. Without further ado, I am excited to introduce Dr Steve Ritter, Welcome to EdTech insiders.
Dr. Steve Ritter:Great to be here. Ben, thanks for inviting me. So first, let's
Ben Kornell:talk about the evolution and impact of AI in education. Carnegie learning has been a pioneer in using AI and instructional software. Could you share how AI integration has evolved at Carnegie learning over the years? Sure,
Dr. Steve Ritter:we're pretty unique in that we started an AI over 25 years ago, and the company really came out of background in cognitive psychology, understanding how people learn and at Carnegie Mellon, their approach in a lot of cognitive psychology was to model build computer programs that mimic human learning and behavior, and in particular, John Anderson's lab would use models to reproduce sort of basic cognitive behaviors on memory and learning. And eventually John was challenged to take that basic research and say, Well, if you can really model how students are learning or how people are learning in general, can you take those models and help them improve instruction? And that was really the origin of the cognitive modeling approach, and in some ways, the origin of AI. So you know, when AI started the Dartmouth conference back in the 50s, was a lot as much about understanding human cognition in order to make computers smart as it was about sort of directly having computers do things that were thought to be things that only humans could do.
Ben Kornell:And for our lay people out there, we hear a lot about neural networks and the attempts to build AI mimicking human thought, what has proven to be true about that approach and what has actually been false, or where we've had to try an alternative methodology?
Dr. Steve Ritter:Yeah, it's a really good question. So for a long time, there's been a split in AI between more symbolic approaches, which, frankly, John Anderson's act R approach is what's considered a symbolic approach. It's rule based largely although there are sub symbolic components that work, like neural networks. And neural networks are more of a statistical approach, taking a whole bunch of data and seeing what you can do with that data. And I think the evolution of AI, particularly over the last 10 years or so, has been to use these statistical approaches, because it turns out, when you have computers that are really fast and that can process a huge amount of data, they can capture statistics that allow them to do like, really amazing things, like we've seen with these large language models like GPT four, there's still open questions about whether what they do, the way they're accomplishing these tasks, has any relationship to what humans do. In some ways they look like it, but there are also like, as everyone who's dealt with hallucinations from a GPT model, often the hallucinations of the character, where it's an error that a human would never make, right? It's just like so far off from what you would do when it's doing things correctly, it seems very human, and when it makes a mistake, it just seems nonhuman. It's like a
Ben Kornell:veneer of comprehension, but then when you poke at it underneath, it's really just a statistical probability dependent on the training data, and there's not like a core understanding or comprehension under
Dr. Steve Ritter:That's right. So there are legitimate questions about maybe that's all humans do is statistical understanding of the training data. But one clue that's I think is really interesting is humans are much more efficient in their learning if you think about the amount of data that these large language models or other neural network based models, the amount of training instances and the amount of data that they receive is really large compared to humans. So humans have a way of generalizing from a relatively small amount of data in a way that tends to work in the world. You know, humans are building hypotheses about the world and testing those hypotheses out in the world, and that becomes like a really powerful way to learn.
Ben Kornell:So this evolution of AI over the last decade that you talked about, and often we talk about generative AI as the kind of most recent version of that. How is that changing the way you approach teaching and learning instruction, course design at Carnegie learning? Yeah.
Dr. Steve Ritter:So generally, I definitely is a game changer. I definitely don't want to be at all dismissive of what it's doing. It gives you really amazing capabilities, in particular to generate language and to lesser extent, to generate images and things like that. I say lesser only because it's really good at illustrations. It's less good at more precise diagramming and the kinds of things that we want to often give students in math and science, but it gives us a lot of expressiveness. So we've been using generative AI to build on top of our cognitive models, so on top of the models that we've built that have like deep understanding about how students think about problems and how students solve problems, and to act as the kind of voice of that knowledge so we can relate to students, because it's really that's the general part. It's really good at producing language that sounds very natural and that's responsive to student inputs. We've also been extending that in the multimodal domain, in thinking. About we have, if you think about like what we've done in mathia, which is the symbolic AI based instructional model that we've been using for a number of years. We looked at last year's data and found that we gave students 3.1 million individual unique feedback messages. Right? Because those feedback messages are generated based on the specific problem that a student's working on and the specific errors or strategies that individual students are using. You couldn't imagine generating 3.1 million videos or animations or diagrams, but sometimes that's the right thing to do educationally, right? And generative AI is now getting to the point where we can think about, you know, in response to a student question, saying, Well, look at this graph right, and it's labeled exactly in the way the student's talking about things, because it's been generated right on a fly. Or here's, you know, a character or a teacher full video to explain it to you, who could maybe gesture on top of that graph and say, like, look at this point here, right? You know, you're confusing the X and Y axes. The point should be here instead of there, and actually point to that. So that's kind of where I see this generative AI going, is the ability to kind of really give students full multimodal feedback, but based on validated and, you know, research based knowledge of the kinds of thinking that students are likely to exhibit and the kinds of strategies that they're likely to pursue.
Ben Kornell:Yeah, it's fascinating. When I was leading a personalized learning company, what we realized is that 80% of the foundation was the same, and the 20% was where the personalization happened. And some of that foundation could be structures or frameworks, some of it could be actual content and knowledge, but this like just, you know, making that last bit customized, responsive, reflective of the learner, was incredible. We were mainly able to measure engagement, and so we were really looking at the engagement differential of that. But now I think the AI is sufficient that you can even start looking at outcomes and the ability for that last mile customization to create differential
Dr. Steve Ritter:outcomes. Yeah, it's a really good observation about personalization, because people do sometimes, like go overboard and think, Well, you know, every interaction is completely unique. But in fact, there are categories just to go back to old school. Ai Herb Simon has this book, the sciences of the artificial, which still holds up really well in a lot of ways. He has this analogy of an ant on the beach. And he says, like, you know, if you want to predict the path of an ant crawling across the sand on a beach, you know, little piles of stand are going to knock the ant left and right. And he basically said, like, you need to know something about ant psychology to predict where the ant goes, but you also, you mostly need to know about the topography of the beach, right where those little hills are. And stuff does a lot. And the same in human problem solving. The nature of the problems that humans are solving dictate a lot about what they're going to do. And so you see a lot of similar behavior. There's differences in that personalization, because there is ant psychology and human psychology, but a lot of it is really about deeply understanding what is the task really for the student, like, what do they need to think about? What are the individual steps they're going to take?
Ben Kornell:Yeah, that's such a great analogy, and it just further emphasizes the need for people who are pedagogic experts to be working alongside technology experts, but also human behavioral psychologists, because there is this sense that the topography is somewhat lost in the excitement of generative AI of the you can go any direction. The best example of this is some of these AI tutor value propositions where it's literally a blank bar. What do you want to learn about? And that's an AI tutor. And I'm like, have these people ever observed a tutoring session that is absolutely not how you would begin. And so I just think there's a real opportunity there. Speaking of great books written about AI, but also about learning, you were a key contributor to the learning engineering toolkit book, and learning engineering is a big part of Carnegie learning's research and their methodology. It's an emerging field. I would say, really, over the last three years, we're just hearing a lot about it, and you've been at the forefront. How do you incorporate learning engineering principles to enhance educational experiences? And maybe even start out with what is learning engineering from your perspective? So.
Dr. Steve Ritter:Yeah, but really excited that learning engineering is getting kind of recognized as a field and a discipline to follow in creating educational experiences. And really what it means is, you know, engineering is the application of science, and so learning engineering is the application of learning science to education, and it emphasizes a lot sort of iterative design, right understanding, you know, as deeply as possible, from the science what the students real learning task is, how do we collect data to understand the specific actions that students are taking to solve problems or to think through some kind of task, and then how do we use that data to help refine the instructional model? So it's very much about thinking about how we put processes in place that are much more likely to produce effective instruction for students. Education is one of these strange fields where teachers get a lot of feedback about what works and what doesn't, but it's on a very local level. They learn for themselves. And just like getting to the point where we can generalize beyond like what happens in that one classroom, and then what that teacher one teacher uses to improve to say, how do we raise the whole field, and I think educational technology in particular has the potential to do that, because now you know, with the technology, and you know the ability to collect data across the internet, you can see beyond a classroom, right? You can see what 10s of 1000s or hundreds of 1000s of students are doing on the same kinds of activities, and just really get a big picture of how that process works?
Ben Kornell:Yeah, in this sense, there's a way in which the kind of neural network, framework of cognition versus the data statistical methods, where you're trying to just capture as much data as possible and crunch it, you could actually see those things coming together, where you have a meaning maker of educator who's able to really come to quite profound conclusions on small data sets, mapped on top of huge data capture, where you're instrumenting classrooms with video and audio as well as written capture. I think that's super exciting. And also why I think, like, assessment is, you know, one of my personal areas where I'm most excited about for the future of AI in education, what are you most excited about? Like, what are some things as you look forward over the next five to 10 years? What kind of areas are you looking for? What kind of predictions or hope fors are on your mind?
Dr. Steve Ritter:Yeah, so I mentioned multimodal feedback, but you can also think about multimodal input and processes so and to follow on your assessment. Mention, assessment is really a strange thing in education, like schools are the only place where we have you. You know, you do things, but what you do, like, if you do projects and whatever, none of that counts. What counts is when you get to the assessment point right where you're sort of not working in a team you're generally working on, you know, they're not necessarily multiple choice questions, but a very constrained type of task that's supposed to display what you learned, right? And what AI holds the potential for is blurring this distinction between learning activities and assessment. And I think that's a big potential, right? So essentially, what we want to do, like if you think about a teacher who asks a student or a group of students to do some kind of project, and if that teacher had the capability to actually watch everything that goes on while students are working on that project, and relate everything that the student is doing to what they should be learning, providing feedback as well, right? That's the best educational experience, and that ought to be the best assessment experience, too, because if you are able to contribute to that kind of project and get to a successful result and learn a lot from it. That's what should count, like, that's the educational experience. And so I do think, like AI, has a strong potential to have us rethink assessment in the sense of incorporating assessment into everyday learning and using that the results of those assessments, both as formative assessments to guide the next activities and things that students should be doing to extend their knowledge, but also as these kind of summative assessments to certify according to kind of a standardized scale, do what standardized tests are doing and say, Yes, this. Student has learned sufficiently that we consider them to have passed an algebra one class, or whatever it is. I
Ben Kornell:think there's this blurring of assessment and learning and basically creating ongoing, real time formative assessment. It's not a new concept. It's been actually around pedagogically, I'm aware since the late 70s, but this idea that now we actually have the tools to instrument it and to do it efficiently reminds me of what you were saying also about the ability to visualize learning. It's not that we aren't trying that tactic. It's just the actual efficiency to be able to do that at scale. So let's talk a little bit about what Carnegie learning is doing at scale and how you're rolling things out. Tell us a little bit about your program and your business. What is the kind of growth trajectory of your work at Carnegie. And you know, for those who want to learn more or find out more, either as a potential employee or teammate or as a potential student, like, tell us a little bit about that programmatic vision. Sure,
Dr. Steve Ritter:like I said, we sort of came out of academic research and how students learn, ended up building these cognitive models that get embedded into our mathia system. But one of the things that we also, I'll say, we learned, maybe we were lucky, but we ended up building a full core curriculum, in part because we thought the software and that what the students were doing in software was really essential to their learning, and that comes from the cognitive psychology you know, practice is a really important component of learning, and practice in particular, in a context where you get good feedback. So what psychologists call deliberative practice, where what you're practicing is kind of well thought out. So that was the idea behind that. We ended up embedding it in a core Mathematics Program, which was really unique in conceptualizing the software as not a supplement to an existing program, but really taking on the whole instructional approach, and that's what's continued for us. Most of our business is about selling math curriculum, including tech, what we call textbooks, but also software. We've expanded beyond math. We now do English, language arts and world languages. And one of the big pushes now is thinking about the reality of full digital textbooks, which is, I'm almost hesitating to use those terms, because once the textbook becomes digital and becomes more than a PDF and more than a digital, you know, reading and testing system. It becomes software just like everything else. And so what we're thinking about now is, where are the boundaries between what we do in mathia, and what we do in what we call our current text materials, you know, it just becomes all activities that are scaffolded digitally, and all of that data becomes available for our recommendation systems. So right now, mathia knows what students do in mathia and makes good recommendations about activities for students, but it's blind to what students are doing outside of mathia. By thinking about this whole full digital solution, we can look at the system more holistically and make choices about, for example, how much should students be working in groups versus individually, right? Instead of prescribing that, that can become a reaction to what we see in the data, a huge issue for teachers is pacing. Every teacher finds themselves, you know, in March or April, saying, oh my god, I can't believe how far behind I am in terms of what I need to cover in this class, and they rush through the end. Well, if you think about monitoring throughout the year, you can start to give pacing recommendations in October, right? That ensure that when March or April comes along, you're not behind, right? You've already kind of planned for how to meet your instructional goals and the time given, rather than sort of doing it in this panic mode towards the end of the school year, I'm really optimistic about a lot of things like that. That's a lot of the effort that we're looking in is how to extend the way that we're looking data to try and improve implementations for teachers and students. Yeah.
Ben Kornell:I mean hearing your response, it also is just a good reminder that the educator in the room is still a really critical partner here in helping learners reach their full potential and just that loop between what the learner is doing what the recommendation engine might suggest, and. Then, you know, informing, supporting and empowering the educator. That loop is a really powerful process. Powerful loop. Yeah,
Dr. Steve Ritter:it's very easy sometimes for people to think, well, AI is going to take over everything, right? And you know, if you spend any time in classrooms, you understand how important the human interaction is to the whole enterprise. Like, Why are students even there, and why should they be listening to the instruction, and why do they care to succeed? You know, all that kind of role is is played by the teacher, and I don't see AI taking over that anytime soon. What we can do is make the teachers more efficient, or help them be more efficient in what they're doing, because computers sort of have this huge memory and huge ability to observe and collect data and stuff like that. But it says an assistant to the teacher and the student,
Ben Kornell:well, a fascinating conversation. Steve Ritter, Carnegie, learning, basically, Carnegie Mellon has seeded some of the greatest edtech companies in the history of education. Carnegie learning one of the superstar companies, and your work foundational to its success in reaching over five and a half million students today. Thank you so much for joining edtech insiders.
Dr. Steve Ritter:Thank you, Ben. This is great
Alexander Sarlin:For our deep dive today. We're here with Chris Kahler. He's the CEO and co founder of Kinnu. Kinnu is a really amazing app for learning, and they're doing some very original things in the space. They just launched Kinnu 2.0 and I'm really excited to have Chris here with us today. Welcome to the podcast.
Christopher Kahler:Hello and thanks for having me. Yeah. So
Alexander Sarlin:first off, for those who might not be familiar with Kinnu, tell us about how it works cool.
Christopher Kahler:Basically, at Kinnu, we build technology and products that accelerate human learning designed around how the brain actually works. I think a lot of edtech and educational products and startups today kind of take how we learn at school as a starting point for how all learning should be, and then iterate on that, either through access or gamification or engagement, whatever. But we wanted to start from first principles by asking what seems like a very obvious question, which is, what is the most efficient way for humans to acquire, retain and use knowledge and build from there and without any preconceptions, and just kind of like see where that takes us. And right now, we have a consumer app with almost a million downloads, and we're beginning pilots with a B to B offering as well. So that's what we do. Congratulations
Alexander Sarlin:on the B to B. I didn't know that that's an exciting expansion, and so I've been a kinder user for a while now, so I can tell a little bit about the experience, but I'd love you to build on this, and especially tell us about what's coming next. You know, when you're in the Kinnu app, you basically have this sort of hexagonal feels like a board game board where you are able to explore these almost like continents of knowledge. You go into each one, it has information and content. It has assessments in line, and you basically, you know, break off bits of the different content and sort of master them, and then move on to others, and all the pieces come together, and you feel like, okay, I'm really acquiring something very new, and it's fun, it's very engaging and addictive as a consumer. But, you know, I think what's so interesting about it is that you have really, really doubled down on the efficacy of it. You really care about it working, about people being able to walk away and actually know more and be able to retain and retrieve that information. So tell us about how you put efficacy at the center of your development process.
Christopher Kahler:Great question, I think, like, We never wanted to build something that was just engaging in fun to use. Like, I think you can build a good edtech consumer business around this principle, but it's not what the founders and I had in mind when we started. Like, we wanted to build something that actually worked. And actually worked means that you have to be able to measure it. So we developed a metric called a K score, which is basically a form of pre post test, and we use, we use that to quantify the efficacy of different features, different content ideas, and kind of hold ourselves accountable so that we know that the experience is actually working. And yeah, that guides product decisions. You know, learning is not just about learning efficiency. It's also about motivation. So sometimes, if we have to make like a motivation trade off, then we can quantify that and say, maybe this feature or this idea like sacrifices a bit of efficiency, but the user engagement is like twice as high. So net, net people learn more, whatever it is. It kind of gives you like, little dials that you can tweak. And our aim is to make this as high as possible. So, like, I kind of, it's a bit tongue in cheek now, but when we first started the company, we really liked the scene from the movie The Matrix. You know when Neo, like, plugs a cable into his head and, like, he says, I know Kung Fu, right? And we call that, okay? So that's time to master equals zero. Is like Neo in the Matrix? Yeah, yep. How do we get time to zero? As close as possible? Possible, given that we can't actually stick things in your brain, and we just have pixels on the screen and sound and haptics and whatever, yeah, and so that's kind of just to give you, like a flavor of thinking. So,
Alexander Sarlin:you know, you put that so well. And I think that that trade off you mentioned, you know, the trade off between engagement and motivation, and sort of the consumer aspects of a mobile app where you want people to engage and be there on a daily basis and have monthly active users, all that great stuff, you know, balancing that with efficiency, especially because, as we all know, people who in learning. You know, learning is not always fun. Sometimes it's hard. Sometimes you have to review things at every time. Sometimes it can be that, you know, they call it productive struggle, that trade off is really intense in edtech, and I think everybody grapples with it, but I think you've taken a really unique approach, because you really put efficacy up front. There's this concept you do called the Virtual Learning Lab that I think could be really, really relevant to others in the field. Tell us about what you do with the Virtual Learning Lab and how you make sure that you really do understand those trade offs. It's not just a theoretical trade off. Let's make something fun that may have an effect. You actually know the effect, because you're actually measuring the learning. Yes.
Christopher Kahler:So basically, what we did was, I mean, like, it was, honestly kind of, it blew our mind, because we were looking for a couple 100 volunteers from the kind of community, and I think over 10,000 people signed up to take part in this. And we're like, Listen, this is going to be a bunch of very hacked together experimental stuff. The experience is going to be crap, but thank you so much. And how it was structured, is it we came up with a bunch of experiments that were inspired from existing science or our own intuitions, or our own research about Feature Ideas, either content specific or engagement specific or FORMAT SPECIFIC, that we thought could move the needle on this case score. And then we did a pre and post test to see if it actually worked, and we compared that against one on one in real life, human tutoring, which we hold to be like the gold standard, where we basically want the same content, which we gave for the tutors. And say, Please make your students learn this. They'll have the same pre test and post test. And you can use any means at your disposal. So, you know, videos, graphs, hand waving, gesticulation, you know, your voice, whatever. And just kind of like, pray that we could get as close as we could to that as possible. And yeah, without being like super hyperbolic, we did like the combination of several feature ideas together outperformed the tutoring for up to high school level stem and humanities pathways. So that was kind of like a moment for us, like internally, we call it like a kind of singularity moment in learning science where, like, holy cow, we should, we should put this stuff in the product. And that's what Kinnu 2.0 which we released a few days ago is all about, yeah, and,
Alexander Sarlin:you know, I just want to hesitate on this and double click on it, because I think this is something that you make it sound very natural here. This is not something that most edtech companies do. They don't. So, you know, my background is, I'm an instructional designer and a product manager. And as an instructional designer, we know that two things that you just said are really important. Pre and post tests are the actual way you measure learning. It's not just about achievement. You have to know where somebody started. You have to have a baseline. And very few edtech companies use that anywhere. They don't use it in their products and they don't use it in their testing. So already kudos. Then you're benchmarking yourself against human tutoring, which is the sort of Bloom's two sigma problem. It's considered the, you know, height of what teaching should and could be one on one. Tutoring is considered the most efficient type of learning there is. So the combination of actually doing pre and post testing, doing it internally, doing with 1000s of users, so you have a high, high end there, and then putting together the benchmarking yourself against such a high standard that's really a sophisticated approach to making sure that the learning is actually happening. So tell us more.
Christopher Kahler:I think if you want to really improve learning, you have to just have some kind of objective measure that it works. Yeah, like, that's really what's the goal here, and we couldn't think of a better way to do it. Like, I think it's also worth saying that tutoring works because it's a person that holds you accountable over a long period of time. That's something not, not to discredit the work of my team or anything, but like, that's something that, like, we obviously can't take into consideration. So this was over like, a shorter period of time, so there's further research here required, but I think it's a starting point. We're pretty excited at the direction of what's possible. Because, you know, tutoring is great if everyone could do it, and the quality of the tutors was the same, and it was like, equally accessible, price wise, for everyone. But it's not right, right? So if we can find a way that can at least, or be as good as that, and do it at scale, we think that that might be able to move the needle, you know, in the ring, which is the
Alexander Sarlin:dream of asynchronous edtech. Let's put it that way, the dream of that doesn't include human tutoring in it, and certainly scalable and low cost edtech. It's incredibly exciting. And then, you know, you mentioned that the experience for these 10,000 learners was hacked together. It was experimental. Is probably MVP prototype kind of features. But do. Getting the results like that led to these, clearly, a lot of product and design sprinting, and there's all sorts of new things. As somebody who's who's used canoe one point, though, I see so many different new features that clearly came out of this research. Tell us about, you know, shields and cranes and some of these really interesting orbs, you know, these concepts that came out of the learning science, out of this research, but then are translated into a very appealing and very consumer friendly design language.
Christopher Kahler:So without belaboring it for people who don't, don't have a reference point for kin at one point, or basically, we needed to come up with a kind of base unit of knowledge that we could track over time so we could measure your retention in the future. Also we will, we will be able to measure mastery, which is coming in probably Kenya, 3.0 which is a one extra dimension. And we need that for building knowledge graphs in the future and a way for you to for us to understand the scaffold of what you know. So we spent a lot of time thinking about this base unit of knowledge, and creating content around that. So that was really cool. It sounds very technical, but as a user, if you learn it's very easy to understand. It's like, this is a core idea. We call them orbs, because concept doesn't really describe it, but this is the core idea. Then you can track your mastery or your memory of that over time. So everything's kind of based around that. The session design is based around that shields are interactive aids to help, to help you memorize that information. Because even though memory has kind of like, it's not very sexy. Now, the research really suggests that you do need to build a scaffold in your mind, and what you know determines what you can learn. Yeah, I think with search and especially with chatgpt, where every like knowledge is at your fingertips, it doesn't replace having stuff in your brain, like we say, for fun, like you can't juggle if you don't have any balls. Yeah, you need balls. So that's one thing. So cranes were inspired by this idea where mastery requires folding 1000 cranes in origami. And this inspired, actually, the entire visual language of the app, which we call like origami. And every time you do a session, you get a stamp, as opposed to a streak, which I think is a very, very in vogue game mechanic that is really good for building a habit, but you feel like crap when you lose it. And it's not really the point. Like learning is a lifelong thing, that where you will fail 100% you will, like, life gets in the way that shouldn't be a reason for you to get, you know, demotivated and throw everything away. So we wanted, like, the game aspect of the design to support this, this concept that, dude, it's cool if you missed a day, you know, like, life's not gonna end. You just have a good work tomorrow. It's gonna be right. And our content engine, where I can talk about this as well. Basically, we use a lot of AI, but we find that for content to really speak to the learner, for it to be creative and in an accessible way, that even GPT four, oh, is not enough. There's much more human touch that's required for the content to really have a life and have a soul. But we're trying to make that not prohibitively expensive, like the human component. And the latest learning engine has what's called storification, which is where we use the material in the pathways and turn it into a store amazing, and have a human touch on top of that. And some of them, like, for example, like, really, really psyched on the Age of Exploration pathway. Like, some personal favorite, now it's just legit good, like, it's really, it reads like a human who cares about this spent a lot of time writing it down, you know, writing it Yeah, yeah. And it's not like, it's half, so it's still, like, AI is really powerful. But I think we're bumping up on, like, the limit, ceiling of how truly great the content can be, and realizing, at least for now, you know, humans are going to be more important than everyone else is. Kind of, is of, is kind of saying, Yeah, we just, like, sounds very counter trend, but we see that also, in the case core like, we've seen that like it quantifiably, it doesn't work as well.
Alexander Sarlin:I don't think it is counter trend because, you know, canoe was ahead of the game in the realization that you could create, you know, mass amounts of high quality, maybe not perfect quality, but if passable and pretty high quality content through AI generation, you were doing that early on in canoe 1.0 and it was, there's a massive expansion from, like, you know, a handful of topics, to dozens and dozens, dozens, and now, I'm sure even more. I think you're probably ahead of the game in realizing that, you know, AI generated content has a lot of strength. Certainly the speed and cost is one of them. But that human aspect, that real, you know, like you say, somebody who cares about the subject, who understands it, who, really, you know, loves it. Let's put it that way. You know, can really, really add something an X factor, something really special there. So you're finding the right ratio. You say it's about half right now, and you're finding, you know, I think that is where the field is going. I think that sort of CO intelligence, to use Ethan Malik's phrase, is exactly where we're headed. It's going to be, you know, pure AI content might read very stiff and and pure human content could read amazingly, but cost a lot, take a lot of time, take a lot of of cycles. So that combination feels very powerful to me. You know, we're in this funny. Moment in edtech, where, like, you know, one of the only public ed tech stocks that has is really been outperforming is Duolingo, right. Pearson's been doing all right, but Duolingo has really been doing well, pretty much continuously for a long time. And one thing I'm excited about about Kendu is it feels like you're taking some of the elements of that Duolingo formula you just mentioned, you know, streaks as one example, the sort of game mechanics that Duolingo has really used, and you're taking it to another level. I really think you're thinking about this stuff more deeply in terms of the actual integrating the learning at the foundation Duolingo is amazing, and they have lots of studies about working in various ways, but at the same time, you know, anecdotally, people wrestle with it, and it's not exactly clear that it's working from from the outside, it feels like you're taking some of that Duolingo, you know, magic, and really taking it to a new level, especially when it comes to that efficacy. Do you look at apps like Duolingo, or apps, you know, some of the other B to C education apps as an inspiration, or do you look at them as sort of a benchmark to surpass?
Christopher Kahler:Great question. I mean, I have so much respect for what Lisa Fanon did with Duolingo and like, it's hard, like, what they did was hard, yeah, and it's definitely that they've cracked engagement and stickiness for a consumer app for like, Who would have thought that, like an app like that could be worth whatever it is, worth today, worth today, on the public market. So definitely, like drew a lot of inspiration. I think the one thing like you said, where we're a bit different is, like the starting point, like they started very much on, how do we build a consumer app that will be super, super sticky people use all the time. If learning happens, that's great. And I think our approach was learning has to happen. If it, if it doesn't happen, it's not good enough for us. We have to start again once we crack that. Like, what are the best ways to get to an engaged because it doesn't have to be as good as dual language engagement wise. Like, this is, like, a super, super high standard. It can, you know, if we had half it would be, like, it would be a total win. Yeah. So, yeah. I think, given the starting points, I think we might end up in in a different place, even though we draw a lot of inspiration from, from how, from their success, yeah, that
Alexander Sarlin:makes sense. It makes a lot of sense. It's really, really exciting. So for those listening, if you haven't already been googling or in the App Store, finding Kinnu, I really recommend you do. I am a user of it, and I am really excited about 2.0 I have not used 2.0 yet, so I'm really pumped about all these new developments. And frankly, as a product person, as an instructional person, instructional design, learning engineer, I am so excited to hear this model of development where you're literally putting efficacy at the center of your process. It's so rare, it's so needed. And I think it could be an inspiration for the whole field. That
Christopher Kahler:would be awesome. Like, there's a lot of work to do, you know, like, even if we fail, we'll do some work that'll inspire the people. Hopefully we won't fail unless other people would join us. I think, yeah, we agree 100% efficacy should be at the core. Yes,
Alexander Sarlin:it's a really exciting model for things, and the design looks beautiful as well. Chris Kahler is the CEO and co founder of Kinnu. It's Kinnu dot XYZ on the web and obviously Kinnu you find it in the app store. It's a really good app. You guys, and Blinkist and a few others are really ones. I come back to often, and you know, just keep on. Keeping on. If you benchmark yourself against tutoring. If you're heading towards mastery, you're combating the forgetting curve, all of those great things you're doing the work. Thanks for being here with us on edtech insiders week in edtech.
Christopher Kahler:Thanks for having me, Alex, it's been a pleasure.
Alexander Sarlin:Thanks for listening to this episode of edtech insiders. If you liked the podcast, remember to rate it and share it with others in the EdTech community. For those who want even more edtech insider, subscribe to the free edtech insiders newsletter on substack you.